
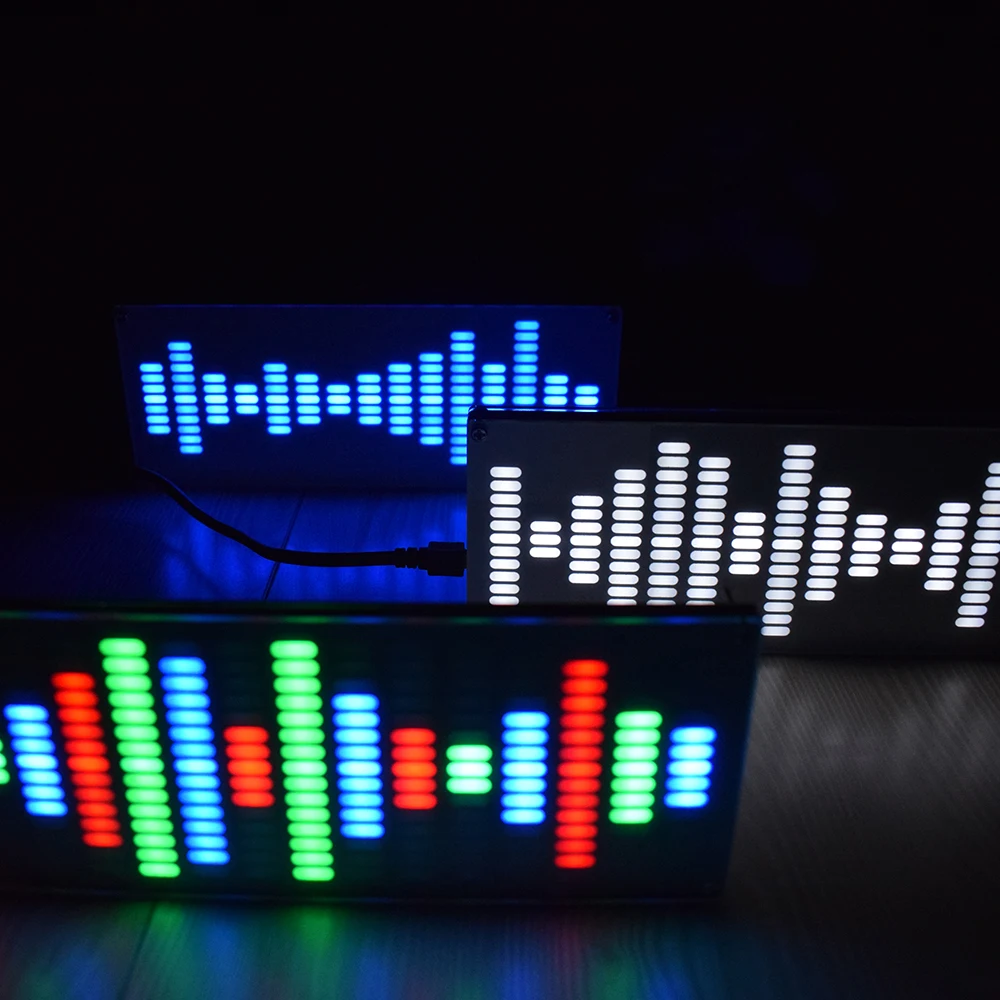
Quantitative and qualitative results show that the performance of our system is comparable to baselines for separation and classification. It was determined that the best configuration for the multi-task learning is to separate the sources first, followed by parallel modules for classification and recovery. Our novel approach exploits the shared information in each task, thus, improving the separation performance of the system. Lastly, it reconstructs the input mixture to help the network further learn the audio representation.
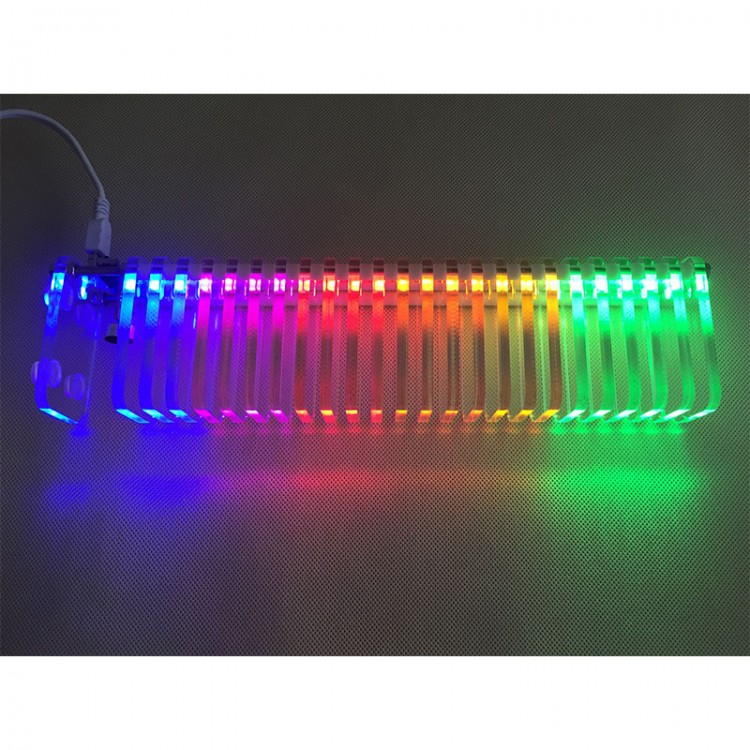
It also detects the presence of a source in the given mixture. The proposed system separates polyphonic music into four sound sources using a single model. In this study, we demonstrate a multi-task learning system for music separation, detection, and recovery. Although these algorithms have proven to have good performance, they are inefficient as they need to learn an independent model for each sound source. Recent studies explored the use of deep learning algorithms for this problem. Music separation aims to extract the signals of individual sources from a given audio mixture. Moreover, we find that combing different front-end features can further improve the system performances. And also on the cross-domain test set, the proposed percussive features (RPFs), and these RPFs with PCEN significantly improve the baseline with conventional log mel-spectrogram features from 81.79% AUC to 84.46% and 88.68%, respectively. Extensive results show that the proposed TCDA leads to a relative 5.02% AUC improvements on mismatch conditions.

Our experiments are performed on the Bird Audio Detection Task of the IEEE AASP Challenge on Detection and Classification of Acoustic Scenes and Events 2018. Furthermore, a harmonic percussive source separation is investigated to extract robust percussive representations of bird call to alleviate the acoustic mismatch. Then, to eliminate the distortion of stationary noise and enhance the transient events, we investigate a per-channel energy normalization (PCEN) to automatic control the gain of every subband in the mel-frequency spectrogram. A time-domain cross-condition data augmentation (TCDA) method is first proposed to enhance the domain coverage of a fixed training dataset. This paper presents front-end acoustic enhancement techniques to handle the acoustic domain mismatch problem in bird detection. Detecting bird calls in audio is an important task for automatic wildlife monitoring, as well as in citizen science and audio library management.
